Package Overview¶
SMAC supports you in determining well-performing hyperparameter configurations for your algorithms. By being a robust and flexible framework for BO, SMAC can improve performance within few function evaluations. It offers several Facades and pre-sets for typical use cases, such as optimizing hyperparameters, solving low dimensional continuous (artificial) global optimization problems and configuring algorithms to perform well across multiple problem Instances.
Features¶
SMAC has following characteristics and capabilities:
- Global optimizer
Bayesian Optimization is used for sample-efficient optimization.
- Optimize Black-Box functions
Optimization is only aware of input and output. It is agnostic to internals of the function.
- Flexible hyperparameters
Use categorical, continuous or hierarchical hyperparameters with the well-integrated ConfigurationSpace. SMAC can optimize up to 100 hyperparameters efficiently.
- Any objectives
Optimization with any objective (e.g., quality or runtime) is possible.
- Multi-Objective
Optimize any number of objectives using scalarized multi-ojective algorithms.
- Multi-Fidelity Optimization
Judge configurations on multiple budgets to discard unsuitable configurations early on. This will result in a massive speed-up, depending on the budgets.
- Instances
Find well-performing hyperparameter configurations not only for one instance (e.g. dataset) of an algorithm, but for many.
- Commandline (CLI)
SMAC can not only be executed within a python file but also from the commandline. Consequently, not only algorithms in python can be optimized but in other languages as well.
Components¶
- Surrogate Models
Gaussian Process
Random Forest (with instances and without)
- Acquisition Functions
Probability of Improvement (PI)
Expected Improvement (EI)
Lower Confidence Bound (LCB)
Thompson Sampling (TS)
- Intensification
Aggressive Racing
Successive Halving
Hyperband
Please see the following figure for a more detailed overview.
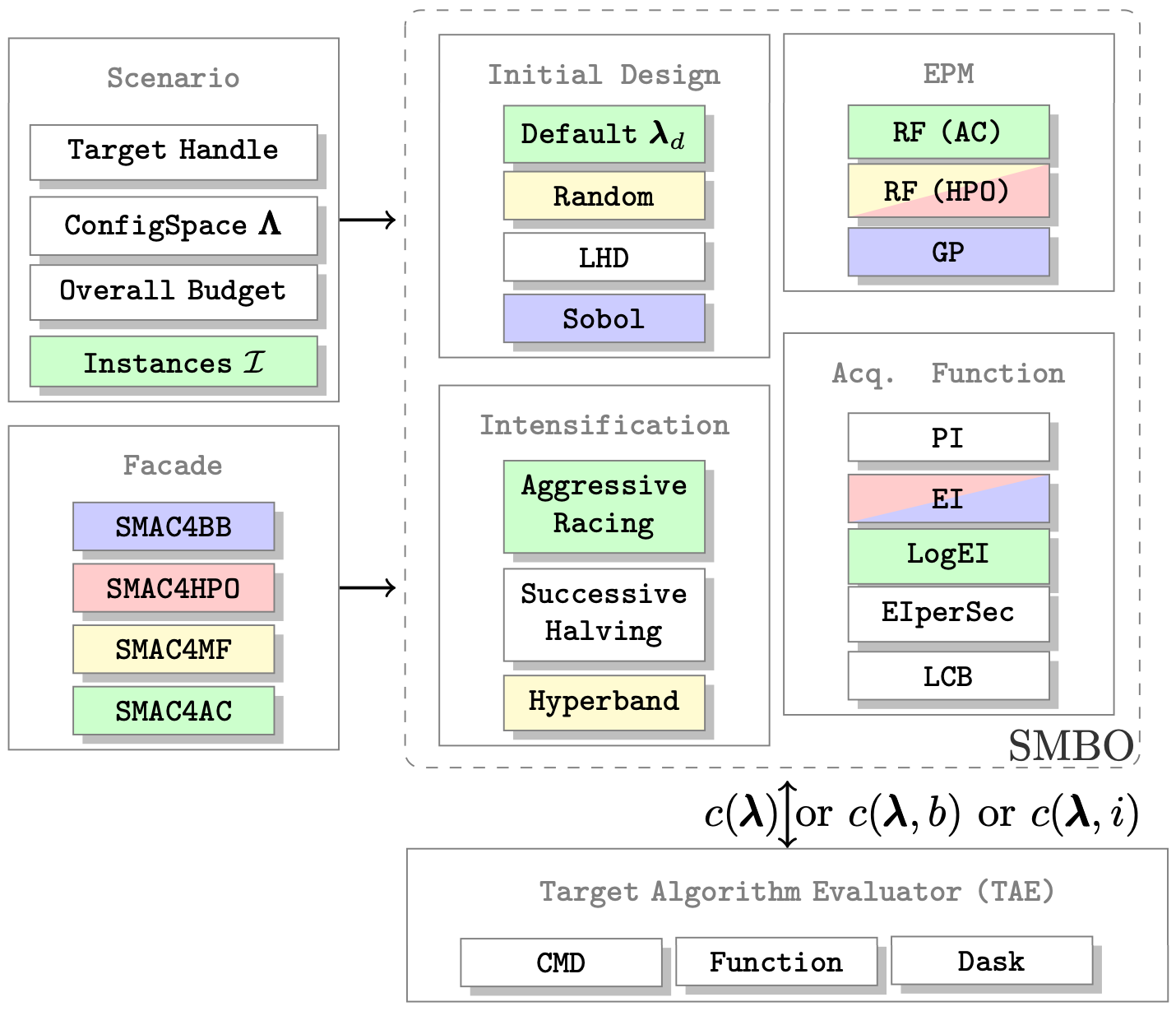
Comparison¶
The following table provides an overview of SMAC’s capabilities in comparison with other optimization tools.
Package |
Complex Hyperparameter Spaces |
Multi-Objective |
Parallelism |
|||
---|---|---|---|---|---|---|
HyperMapper |
✅ |
✅ |
❌ |
❌ |
❌ |
❌ |
Optuna |
✅ |
✅ |
❌ |
❌ |
✅ |
✅ |
Hyperopt |
✅ |
❌ |
❌ |
❌ |
✅ |
✅ |
BoTorch |
❌ |
✅ |
✅ |
❌ |
❌ |
✅ |
OpenBox |
✅ |
✅ |
❌ |
❌ |
❌ |
✅ |
HpBandSter |
✅ |
❌ |
✅ |
❌ |
❌ |
✅ |
SMAC |
✅ |
✅ |
✅ |
✅ |
✅ |
✅ |